Since Apple introduced ATT (App Tracking Transparency) and SKAdNetwork with iOS 14.5, back in March 2021, the app marketing ecosystem has been changing dramatically. One consequence we want to discuss and highlight in this article is the absence of lookalike audiences for app install campaign optimization.
Lookalike audiences are audiences generated by algorithms using data from a small set of users, such as the one provided by a CRM system. Once there is an audience, created by using device IDs, algorithms expand this set to generate larger audiences based on the same signals. Lookalike audiences help increase the app install campaign reach and improve the conversion rate. The idea behind lookalike audiences is to create an audience which reflects your high-quality customers, in order to run app install campaigns targeting users with similar characteristics.
However, Apple’s new regulations about privacy have made it extremely difficult to create lookalike audiences because iOS users can now decide not to share their unique device identifier (IDFA). Without IDFA, it’s almost impossible to create audiences and even more difficult to expand them to lookalike audiences through algorithms. So what’s left for ad networks and mobile DSPs to optimize paid user acquisition campaigns ?
Well, a lot actually, and let’s see how to leverage machine learning algorithms in order to carry out the app campaign optimization contextually, without using device IDs.
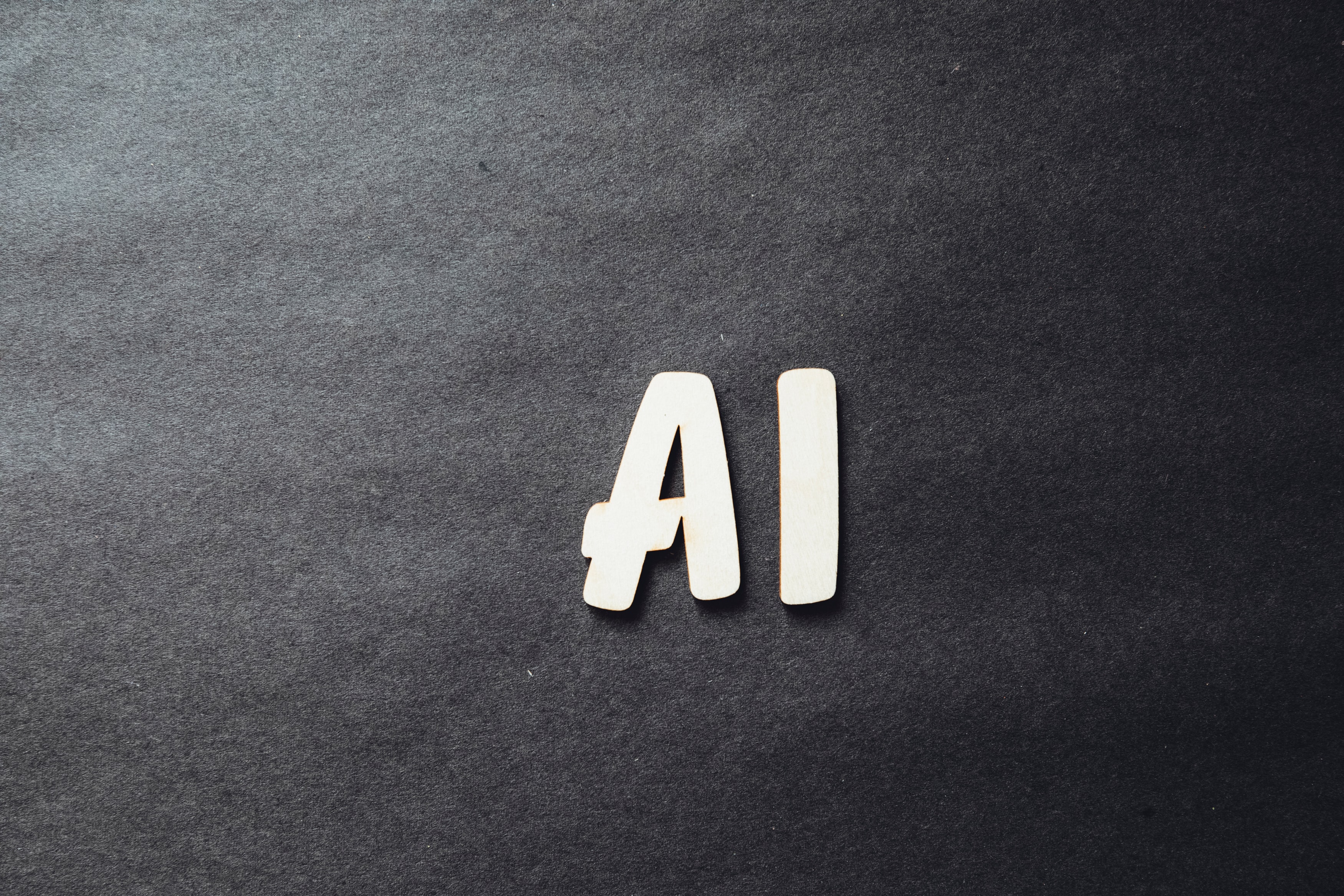
Contextual Optimization In The Post-Audiences Era
First of all, please remember that lookalike audiences are a tool for app campaign optimization in order to achieve specific KPIs set by the advertisers. In-app optimization is an example of what app marketers do in order to increase the number of in-app events generated by the app campaign. Therefore, the first step is still to set clear and measurable KPIs, which can be the install volumes, the app campaign ROI, the retention rate and so on.
Once the KPI is crystal clear, app marketers can start running app campaigns at full steam, leveraging all of their tools for the optimization process.
After starting the app install campaigns with the targeting criteria provided by advertisers, mobile DSPs and mobile Ad Networks can analyze the data collected. That’s when machine learning algorithms come into place. Indeed, ML algorithms are able to analyze huge amounts of data that are impossible for the human brain alone to digest.
Speaking about app campaigns, ML algorithms are frequently used to analyze the traffic acquired by monitoring multiple contextual signals at the same time. For example, they can monitor:
- Operating system;
- OS version;
- Internet connection;
- Country/State;
- City (even at the ZIP code level);
- Hour of the impression/click/conversion.

These are only some of the data processed by machine learning algorithms during the traffic analysis. The next step is to create pockets of traffic by combining the contextual signals and then assess those pockets in terms of their quality, which is how they work in generating the desired results.
Some pockets are better than others in generating a high click-to-install rate or in driving in-app purchases, which help increase the campaign ROI and users’ LTV. As a result, ML algorithms will start to buy traffic from the best performing pockets based on the desired KPI, improving the overall performance of the app install campaigns.
However, it’s important to note that this must be an iterative process, because the more data ML algorithms ingest the more they are able to refine the analysis and lead to better results. This means testing new sources but also buying traffic also from existing pockets outside the best ones, in order to assess their performance and explore more data.
To Sum Up
This is just an example of how machine learning algorithms can help app marketers to run their app install campaigns with excellent results, based on our experience. Even though it’s almost impossible to create lookalike audiences, DSPs and Mobile Ad Networks can leverage many other resources such as A.I. and ML algorithms in order to acquire high-quality users and generate valuable results for advertisers.